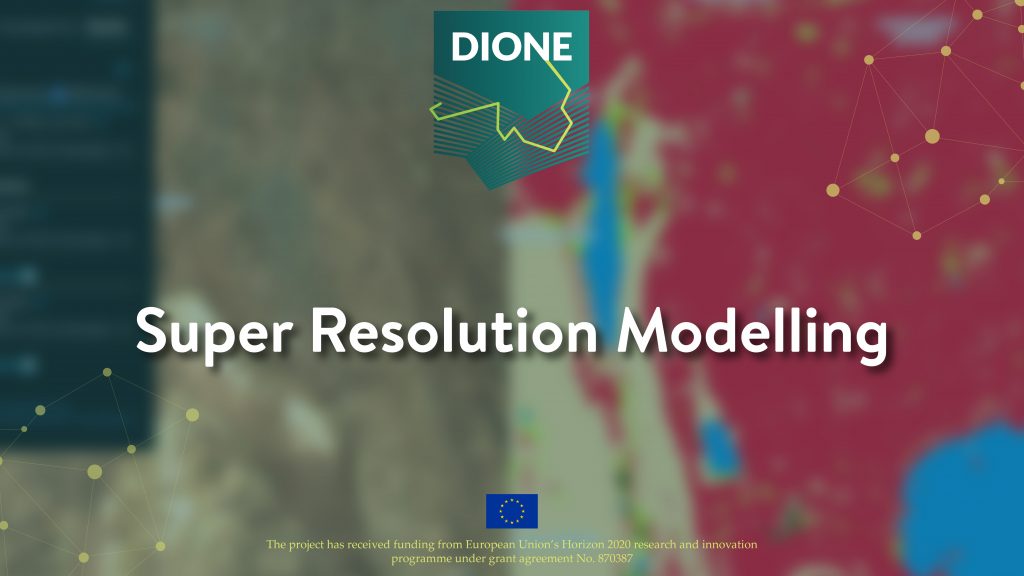
Super Resolution is the process of recovering a High Resolution (HR) image from a given Low Resolution (LR) image. An image may have a “lower resolution” due to a smaller spatial resolution (i.e. size) or due to a result of degradation (such as blurring).
Development of a super resolution model starts with the preparation of the training dataset. Very high resolution (VHR) imagery (Deimos in the DIONE Project example) is used as labels to the model. This data needs to be preprocessed in such a way as to be suitable for model training (cloud free, normalizations etc.). The features of the model are temporally stacked Sentinel-2 images within two months of the Deimos acquisition. The model used is HighRes-Net, which was one of the best performing models in ESA’s Kelvin competition for super resolution Proba-V imagery. Once the model is trained, the prediction is run over areas in Lithuania and Cyprus. The resulting imagery is then ingested into Sentinel-Hub where it can be requested by other applications.
In our case, Super Resolution Modelling tries to improve the spatial resolution of Sentinel-2 imagery by teaching a deep neural network to upscale images. This is done by showing the model temporally stacked Sentinel-2 imagery as features and very high resolution imagery over the same area as labels. This allows the model to map the Sentinel-2 low resolution representation into a higher resolution representation. This increases the resolution of the images from 10m to 2.5m while retaining the temporal resolution (we can super-resolve all Sentinel-2 images during a certain timeframe), the advantage for DIONE is that this should enable monitoring of smaller parcels (up to 100m2) without the need for a large amount of expensive VHR imagery.
For more news follow the DIONE web presentation.